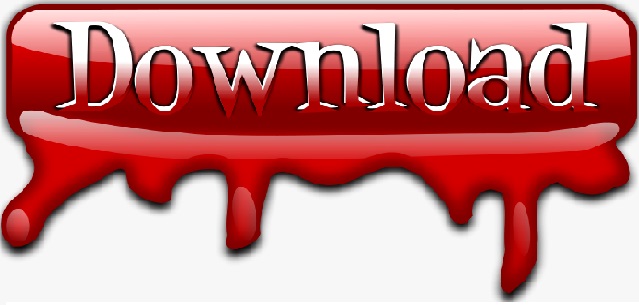

However, significant barriers exist during biologists' conventional miR knowledge discovery. miRNAs or miRs) have been reported to perform important roles in various biological processes by regulating respective target genes. Thus, the entire process, which is fully automatic, transform facts embedded within tables into facts accessible by standard query engines.Īs a special class of short non-coding RNAs, microRNAs (a.k.a. We further show that semantically annotated data leads immediately to queriable data. Labels in nested table structures yield ontological concepts and interrelationships among these concepts, and associated data values become annotated information. Further, given that we can automatically interpret tables, we next show that this leads immediately to a conceptualization of the data in these interpreted tables and thus also to a way to semantically annotate these interpreted tables with respect to the ontological conceptualization. For these activities, the system was able to achieve an overall F-measure of 94.5%.

Experimental results show that the system can successfully identify sibling tables, generate structure patterns, interpret tables using the generated patterns, and automatically adjust the structure patterns as it processes a sequence of hidden-web pages. We tested our solution using more than 2000 tables in source pages from three different domains-car advertisements, molecular biology, and geopolitical information.

Our system compares them to identify and connect nonvarying components (category labels) and varying components (data values). The sibling pages we consider are pages on the hidden web, commonly generated from underlying databases. In this paper, we offer a solution for the common special case in which so-called sibling pages are available. Its solution would not only be an aid to table processing applications such as large volume table conversion, but would also be an aid in solving related problems such as information extraction, semantic annotation, and semi-structured data management. The longstanding problem of automatic table interpretation still eludes us.

It takes as input the couple of key and value of an attribute of a JSON object, the attribute links it to the object that contains it (p_attrib_name), the value of its identifier (indivname), domain_cl and domain_indiv. keys() do 3 if key ∈ pr imary_keys then 4 id ← key cr eat eCl ass(p_at t r ib_name) 5 domain_cl ← f indCl ass By N ame(p_at t r ib_name) 6 end if 7 end if 8 type ← type(at t r ib_value) 9 // The type function allows us to determine the type of the attribute value 10 if type ∈ basic_types then 11 cr eat e Dat aP r oper ty(at t r ib_name, domain_cl, type) 12 inst ant iat e DP (domain_cl, indivname, at t r ib_name, 13 at t r ib_value) 14 else if type is List then 15 for it em ∈ at t r ib_value do 16 at t r ibT oOnto(at t r ib_name, it em, p_at t r ib_name, 17 indivname, domain_cl, domain_indiv) 18 end for 19 else 20 // Dictionary type 21 ob jpr op_name ← has + at t r ib_name 22 r anдe_cl ← f indCl ass By N ame(at t r ib_name) 23 if r anдe_cl = N ull then 24 cr eat eCl ass(at t r ib_name) 25 r anдe_cl ← f indCl ass By N ame(at t r ib_name) 26 end if 27 ob jpr op ← f ind P r opBy N ame(ob jpr op_name) 28 if ob jpr op = N ull then 29 cr eat eOb ject P r oper ty(ob jpr op_name, domain_cl, r anдe_cl ) 18) or dictionary type (line 19 to 37). The matching method is based on the type of the value of the key: basic (e.g., integer, string, float, Algorithm 1: JSONObjectToOnto Input : json_ob ject, cl ass_name, domain_cl, domain_indiv Output : indivname 1 id ← N ull 2 for key ∈ json_ob ject.
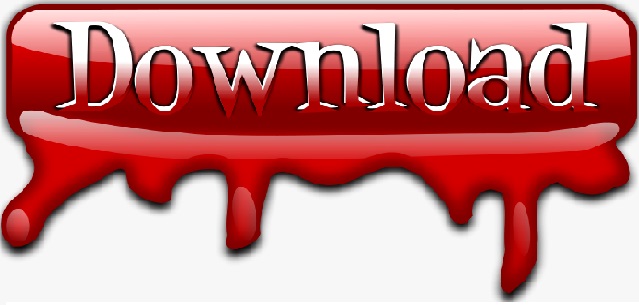